class: center, middle, inverse, title-slide # Variable selection for causal inference ## What If: Chapter 18 ### Elena Dudukina ### 2022-03-23 --- # 18.1 The different goals of variable selection - No adjustment needed when the aim is prediction - Include any variables that improve predictive ability - Automated variable selection for prediction models - Lasso and other regression algorithms - Non-regression algorithms, e.g. neural networks - Use cross-validation to fine tune predictive accuracy - Adjustment for confounding and other biases when the aim is causal answer - Automated approaches for variable selection are arguably less appropriate due to the risk of introducing bias - Subject matter knowledge --- # 18.2 Variables that induce or amplify bias .pull-left[ - Unbiased causal effect estimation needs no adjustment - Adjusting for `\(L\)` creates selection bias under the null ] .pull-right[ 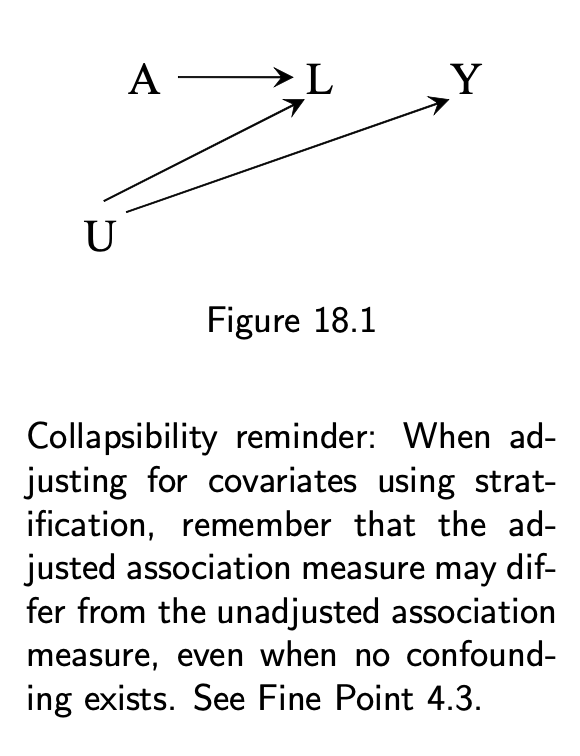 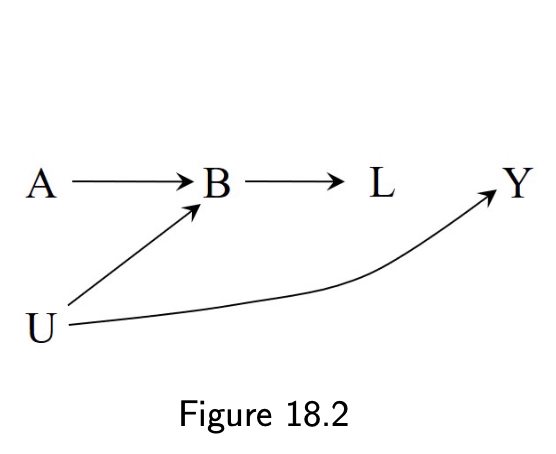 ] --- # 18.2 Variables that induce or amplify bias .pull-left[ - Selection bias under an alternative ] .pull-right[ 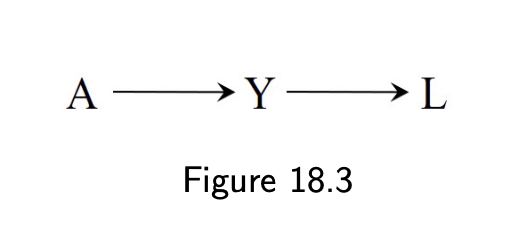 ] --- # 18.2 Variables that induce or amplify bias .pull-left[ - Overadjustment for a mediator (18.4) - Adjust for post-treatment L (18.5) ] .pull-right[ 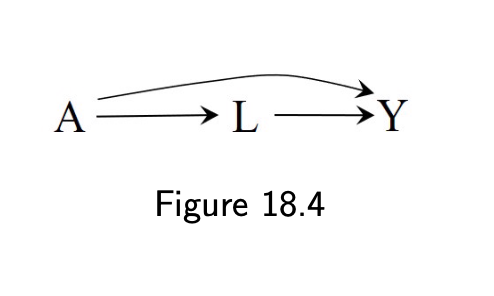 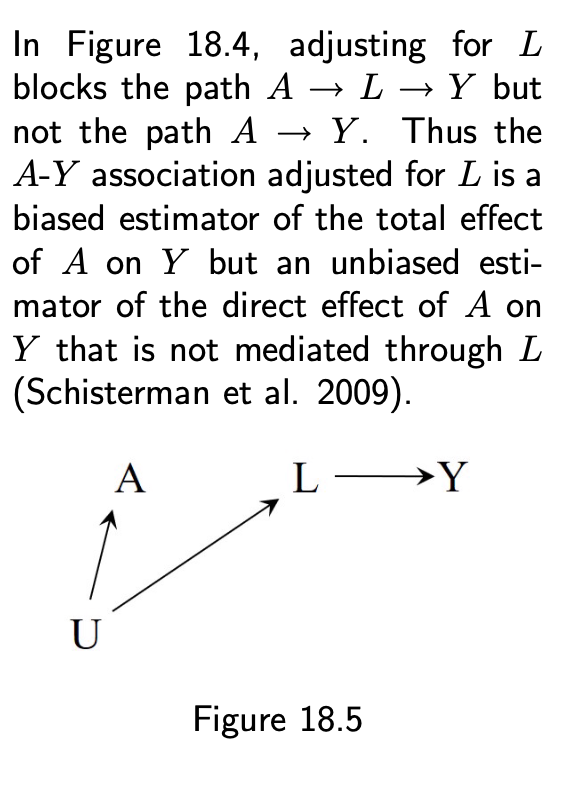 ] --- # 18.2 Variables that induce or amplify bias .pull-left[ - M-bias - L is not a confounder ] .pull-right[ 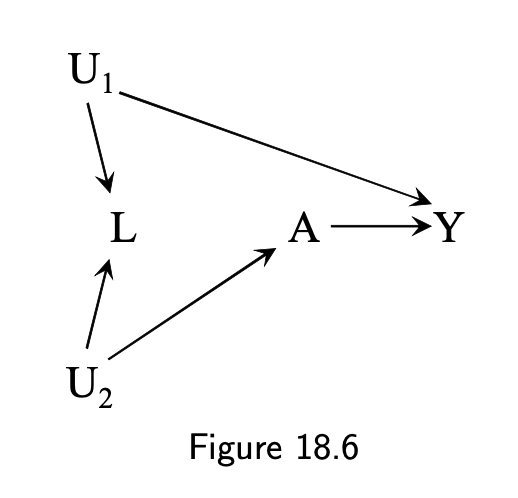 ] --- # 18.2 Variables that induce or amplify bias .pull-left[ - Bias amplification when adjusting for instrument Z ] .pull-right[ 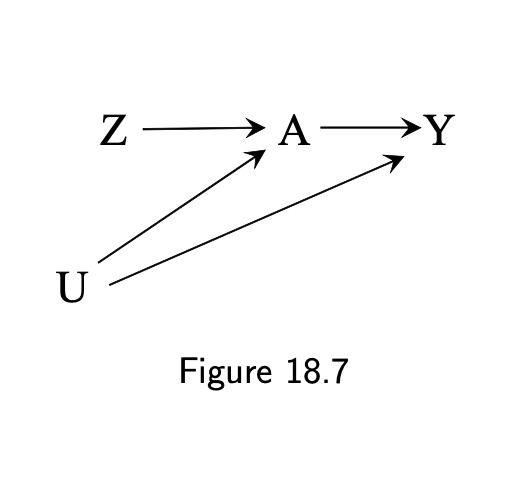 ] --- # 18.3 Causal inference and machine learning - ML algorithms do not guarantee that the selected variables eliminate confounding - Doubly robust estimator gives smaller bias and smaller, however the variance of the estimate may be wrong - Wrong coverage: fail to trap the causal parameter of interest at least 95% of the time - ML algorithms are statistical black boxes with largely unknown statistical properties --- # 18.4 Doubly robust machine learning estimators - Split the sample - Apply the predictive algorithms to the training sample in order to obtain estimators - Compute the doubly robust estimator of the average causal effect in the estimation sample using the estimators from the training samples --- # 18.5 Variable selection is a difficult problem - > Doubly robust machine learning does not solve all problems - > Available subject-matter knowledge are insufficient to identify all important confounders or to rule out variables that induce or amplify bias - > No algorithm is optimal in all settings - > Implementation - > No guarantee that estimate's variance will be small enough for meaningful causal inference - > Tension between including all potential confounders to eliminate bias and excluding some variables to reduce the variance is hard to resolve - Developing a clear set of general guidelines for vari- able selection may not be possible --- # References 1. HernĂ¡n MA, Robins JM (2020). Causal Inference: What If. Boca Raton: Chapman & Hall/CRC (v. 30mar21) 1. A Crash Course in Good and Bad Controls [paper](https://www.datascienceassn.org/sites/default/files/A%20Crash%20Course%20in%20Good%20and%20Bad%20Controls.pdf) 1. A Crash Course in Good and Bad Controls [blog](http://causality.cs.ucla.edu/blog/index.php/category/bad-control/) 1. Good & Bad Controls [Statistical Rethinking 2022 Lecture 06](https://www.youtube.com/watch?v=NSuTaeW6Orc) 1. Econometrics: Control Variables [video](https://www.youtube.com/watch?v=Ba2Nhn4co88) by Nick Huntington-Klein 1. Targeted Learning in R: Causal Data Science with the [tlverse](https://tlverse.org/tlverse-handbook/index.html#about-this-book) Software Ecosystem